Fisher's exact test is a statistical significance test used for small sample sizes. It is one of a number of tests used to analyze contingency tables, which display the interaction of two or more variables. This test was invented by English scientist Ronald Fisher, and it is called exact because it calculates statistical significance exactly, rather than by using an approximation.
To understand how Fisher's exact test works, it is essential to understand what a contingency table is and how it is used. In the simplest example, there are only two variables to be compared in a contingency table. Usually, these are categorical variables. As an example, imagine you are conducting a study on whether gender correlates with owning pets. There are two categorical variables in this study: gender, either male or female, and pet ownership.
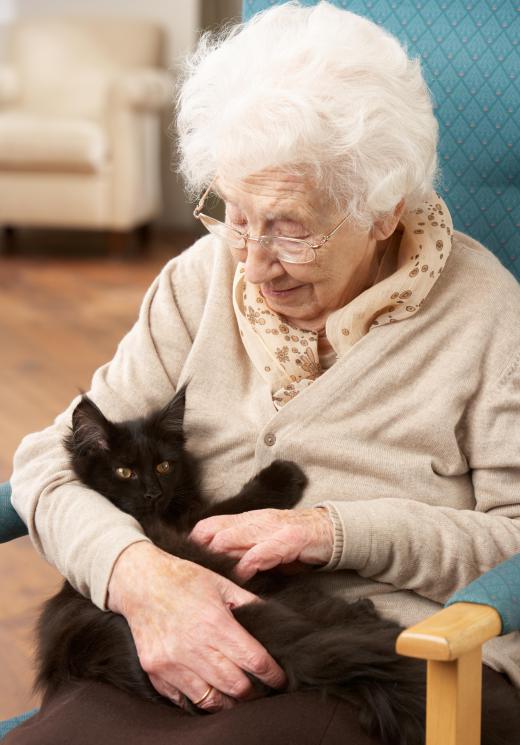
A contingency table is set up with one variable on the top, and the other on the left side, so that there is a box for each combination of variables. Totals are given on the bottom and at the far right. Here is what a contingency table would look like for the example study, assuming a survey of 24 individuals:
Pet Owner | Not a Pet Owner | Total | |
Male | 1 | 9 | 10 |
Female | 11 | 3 | 14 |
Total | 12 | 12 | 24 |
Fisher's exact test calculates deviance from the null hypothesis, which holds that there is no bias in the data, or that the two categorical variables have no correlation with each other. In the case of the present example, the null hypothesis is that men and women are equally likely to own pets. Fisher's exact test was designed for contingency tables with a small sample size, or large discrepancies between cell numbers, like the one shown above. For contingency tables with a large sample size and well-balanced numbers in each cell of the table, Fisher's exact test is not accurate, and the chi-square test is preferred.
In analyzing the data in the table above, Fisher's exact test serves to determine the probability that pet-ownership is unevenly distributed among men and women in the sample. We know that ten of the 24 people surveyed own pets, and that 12 of the 24 are female. The probability that ten people chosen at random from the sample will consist of nine women and one man will suggest the statistical significance of the distribution of pet owners in the sample.
Probability is denoted by the letter p. Fisher's exact test determines the p-value for the above data by multiplying the factorials of each marginal total -- in the table above, 10, 14, 12, and 12 -- and dividing the result by the product of the factorials of each cell number and of the grand total. A factorial is the product of all positive integers less than or equal to a given number. 10!, pronounced "ten factorial," is therefore equal to 10X9X8X7X6X5X4X3X2X1, or 3,628,800.
For the table above, then, p= (10!)(14!)(12!)(12!)/(1!)(9!)(11!)(3!)(24!). Using a calculator, one can determine that the probability of getting the numbers in the table above is under 2%, well below chance, if the null hypothesis is true. Therefore, it is very unlikely that there is no contingency, or significant relationship, between gender and pet-ownership in the study sample.